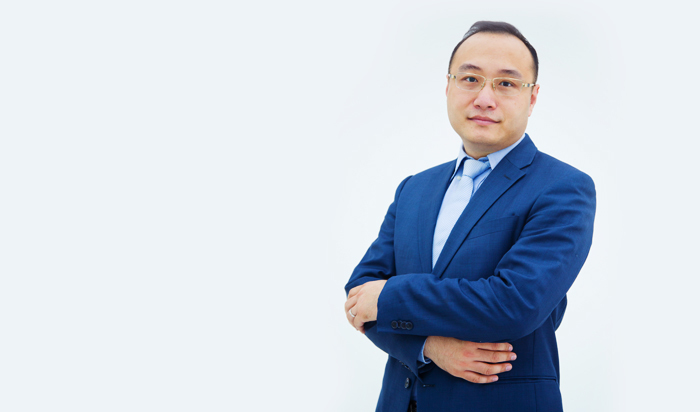
In a span of about three years, Huiyihuiying (HY) has become a leading company in the development and implementation of AI in the medical sector in China. The enterprise, which focuses on AI for medical imaging, recently launched a new product at the Radiological Society of North America’s Annual Meeting (RSNA 2018), which can intelligently screen for tuberculosis and quantify the location and shape of tuberculosis texture by combining X-rays and CT analysis.
In an email interview with Healthcare IT News Asia Pacific, Xiangfei Chai, CEO and founder of HY, shared on his journey behind starting the company, some observations in the key trends in the developments of AI technologies in healthcare within China and abroad, as well as some of the obstacles in the developments of AI in healthcare.
You have been a medical imaging researcher and developer for almost a decade, working in the department of radiotherapy/radiology at well-known academic hospitals. How did the idea to start Huiyihuiying (HY) in 2015 come about?
Since my time as a graduate student, I have been with the hospital and also working with medical image industry for more than ten years. I had been involved in the development of imaging applications, which includes guided radiotherapy systems, image cloud platform, radiotherapy cloud platform, etc. in the Netherlands Cancer Research Centre and the Stanford University School of Cancer Radiotherapy Centre.
I may have continued my post-doctoral and research working in the medical imaging field if I didn’t start the business. If so, this is how I see my life will be like decades later.
The laboratory is the cradle of AI. Stanford University is the cradle of AI entrepreneurs and the main battlefield of the global artificial intelligence. For a long time, Stanford University has a very good environment that fosters innovation and entrepreneurship, encourages bold ventures, with a freedom to explore atmosphere and multiculturalism that tolerates failure. For example, teachers can manage one day a week freely that does not require them to engage in school teaching and research. They are allowed to work as a consultant or an independent director.
How to turn scientific research results into use results is what I want to verify from the postgraduate era. Although it is not easy to productise and commercialise the theory, it is worthwhile to do so.
At the beginning of 2015, I left the Stanford University Medical College Affiliated Hospital and ended my 12-year medical imaging academic career. I founded Huiyihuiying (HY) and wanted to explore further.
HY recently launched their new AI Full Cycle Health Management Cloud Platform, which consists of two separate platforms for different health concerns: the Breast Cancer AI Full Cycle Health Management Platform and the AORTIST 2.0 Aorta AI Cloud Platform. Both platforms are based on the AI 2.0 technology. Could you tell us what AI 2.0 tech is in a nutshell and its main advantages over ‘conventional’ AI?
For AI1.0, we use Convolutional Neural Networks (CNN), Fast Region-based CNN (RCNN), Residual Networks (ResNet) and other technologies to identify lesions, assist imaging and screening diagnosis, improve the efficiency of images for doctors and reduce misdiagnosis, which is the solution for main AI products. An example would be AI lung nodule screening applications.
AI2.0 is based on image data, clinical data, pathological data, etc., combined with follow-up information, we use natural semantic recognition technology, use AI to empower the whole process of medical treatment, from pre-diagnosis to participation in treatment decision-making, prognosis prediction and follow-up monitoring to achieve evidence-based medicine. At present, some of the operations in many top hospitals are prosthetic ones with high proportion of postoperative recurrence.
Prognosis prediction and follow-up is a challenge of this type of complex disease. We are targeting to design a patient-centered product that covers the patient’s entire medical cycle. Besides improve the surgeon’s surgical accuracy, the AORTIST system integrates the radcloud platform developed by HY and embeds a prognostic prediction model that will provide the prediction after surgery of B-type dissection.
What are some key trends that you observe in the developments and applications of AI in healthcare in China and more broadly, world-wide?
Patient-centred applications are promising. Since 2010, improving patient experience has become the mainstream of the US medical community. We believe that the ultimate goal of both doctors and patients is the same that is to cure the disease. So we adjusted the entire product design logic to patient-centered six months ago to improve the patient experience.
Entering the era of data-driven precision medicine: From 1898 onwards, we have experienced the era of physical driven represented by X-ray, ultrasound, nuclear magnetic, etc., and application driven represented by image guidance and treatment plans. After 2010, we have entered the era of data-driven precision medicine. Its typical feature is to mine effective information in massive data and optimise diagnosis and treatment methods.
Artificial intelligence participates in the medical cycle management: In many complicated diseases, prognosis prediction and follow-up are big challenges. AI can be integrated with multi-dimensional data such as imaging, genetics, pathology and clinical, to provide individual medical solutions for patients, recommend surgical plans for clinicians and provide medication guidance.
AI can play a greater value in the medical cycle by providing patients with reasonable examination, treatment, follow-up and rehabilitation programmes, provide comprehensive monitoring and management of the entire disease, optimise the diagnosis and treatment process and reduce medical expenses overall.
What do you feel are obstacles or roadblocks to AI development in healthcare?
First of all, compared with US-European countries, there is a large number of interdisciplinary talents especially in the medical imaging AI industry which is an interdisciplinary industry. Therefore, it needs diverse and interdisciplinary portfolio with both technical and marketing teams. With that, people with different knowledge and experience backgrounds can gather wisdom in different fields and eventually form a closed loop of productivity that can break through the limitations of a single discipline. The reality is that doctors have a relative lack of understanding of technology and it is difficult for technical talents to have a deep understanding of the medical field.
Second, data is the key. Medical big data is very special that it doesn’t have big volume, even image data is very limited, especially in a single disease. Normally each of us do not even take one film scan per year, such as for interstitial pneumonia or fractures. There are only several thousands of patients in the country every year and they are scattered in various hospitals. Data acquisition is very difficult. In addition, the data collection standards between hospitals are not uniform and there is a large amount of unstructured data.
Third, in the development and deployment of AI applications, there are different brands and models of equipment used in different hospitals, resulting in differences in image layer thickness, layer spacing, etc., there is a need to optimise the image and normalise the processing to ensure the validity of the data. It is also necessary to interface with the existing data systems of the hospital according to the specific conditions of the hospital to ensure the stability and safety of the operation.
Fourth, this is a Chinese characteristic – the demand and supply of medical resources in China has long been an unbalanced “mismatched” situation. In the context of the Chinese government’s implementation of grading diagnosis and treatment, artificial intelligence applications have entered medical care, especially the grassroots also face some fundamental problems and medical informationisation has become a rift in the field of artificial intelligence.
Although there are many Chinese medical information companies, the standards are not uniform, including all interfaces, specific implementation of each hospital and each hospital has done a lot of personalised localisation improvements which leads to great progress in medical informationisation. The direction is more structured, more standardised and more unified. Informatisation solves not only the efficiency problem, but also makes the overall information flow better form the basis and data source of artificial intelligence.
HY is collaborating with more than 800 medical institutions in China in clinical applications and scientific research projects, including the Chinese PLA General Hospital, Peking Union Medical College Hospital, Beijing Friendship Hospital and several medical associations. The company also plans to expand its business to the other parts of the world – what are HY’s plans for the Asia-Pacific market?
Huiyihuiying is actively developing overseas markets and has set up branches in the United States. Currently, we are covering Japan, France, Kazakhstan, the United States, India, Israel, etc. For example, we signed a contract with Kazakhstan’s largest private hospital chain group, established cooperation with Japan’s largest cloud PACS company on radcloud platform, cooperated with France largest oncology company and developed US market with US medical AI companies, etc.
In the future, besides strengthening cooperation with countries along the “Belt and Road” initiative, HY will collaborate with more partners around the world and strive to make medical AI another beautiful business card in China.
In a relatively short period of about 3 years, HY has emerged to become a leading company in the development and implementation of AI in the medical sector. What do you think are some of the main factors for HY’s success and what do you hope for HY to achieve in the long-term?
First of all, it is very important to condense a large number of outstanding interdisciplinary talents. HY is constantly improving the introduction and training mechanism of outstanding talents.
Second, medical treatment is a very complicated matter, especially medical AI. It is not a single breakthrough. HY is building a team culture where everyone is a product manager. Everyone is a team manager of customer managers, able to bring products, technology, sales are always in sync and balanced.
Third, HY has established a full-cycle data intelligence platform to build a full-cycle, high-value database with large hospitals through NLP intelligent extraction, structured reporting, and intelligent follow-up. High-quality data is based on the labeling of a large number of professional doctors. HY uses three-blind labeling instead of double-blind labeling. Each case is marked by at least 3 professional imaging doctors. We have obtained millions of cases.
Fourth, we adopted migration learning last year. We combined image data with clinical data, test data, and genetic data on a self-built full-scale data platform to build AI models in multi-dimensional data to achieve small data sets. Accurate modeling on the surface overcomes many problems of disease dispersal and less complete data, ensuring good model training results.
Lastly, in terms of computational power, we take the lead in using Intel’s EXON scalable processor to enable its latest scalable computational resources to converge into the medical image, which surpasses the memory limitation of GPU and it can conduct unsupervised learning on three-dimensional CT and MRI data and U-Net segmentation without manual labeling data, directly use PACS and RIS data to score that greatly improves the efficiency of modeling.
In the future, we hope to break through the barriers of data, combine genomics, proteomics, molecularomics, metabolomics and imaging-omics, etc. to build a full-scale data centre and then model, mine the greater value behind the data, assist clinical decision-making and promote personalised diagnosis and treatment. This is the biggest vision of my ten years and one of our biggest dreams.