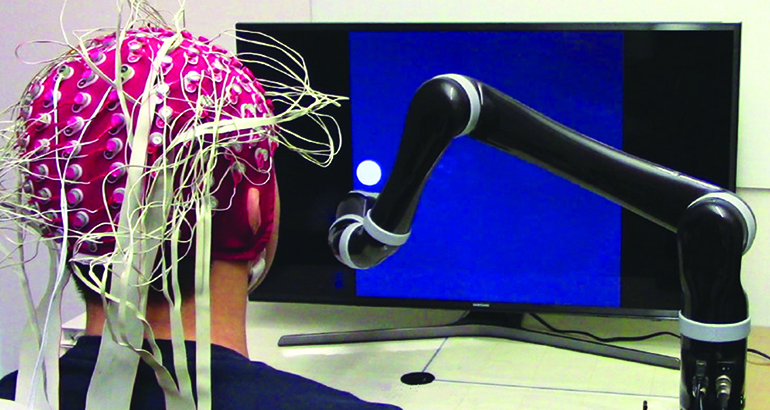
Scientific collaborators from Carnegie Mellon University and University of Minnesota have created a way for people to control a robotic arm using a non-invasive brain-computer interface (BCI). Previously, electrode array implants in the brain have been necessary to give severely disabled people the ability to manipulate an external robot. That is because implants can gather more actionable signal information by being placed right on the surface of the brain. Avoiding dangerously invasive brain surgery to place these implants, though, is a big goal in the field of brain-computer interfaces.
The Carnegie Mellon team turned to newly developed sensing and machine learning methods to accurately read signals coming from deep within the brain, relying only on an external electroencephalography cap for signal gathering. The system can quickly improve both its performance and that of the person using it, to achieve drastically better results than previous solutions. Volunteers using the technology were put through a pursuit task and a training regimen to improve their engagement, while the system was performing an analysis of their brain signals.
Here’s a video showing how the system allows for accurate, and very smooth tracking of a cursor on a screen by a robotic arm that’s mind-controlled by a human:
Some impressive findings have emerged from the study of the technology appearing in journal Science Robotics:
In all, our unique framework enhanced BCI learning by nearly 60% for traditional center-out tasks and by more than 500% in the more realistic continuous pursuit task. We further demonstrated an additional enhancement in BCI control of almost 10% by using online noninvasive neuroimaging. Last, this framework was deployed in a physical task, demonstrating a near-seamless transition from the control of an unconstrained virtual cursor to the real-time control of a robotic arm.
Study in journal Science Robotics: Noninvasive neuroimaging enhances continuous neural tracking for robotic device control